Yongxin Chen’s research combines the power of machine learning, control, and robotics.
AI's integration into our daily lives is undeniable, whether it's seeking answers from ChatGPT or the increasing number of self-driving cars on the roads. Its widespread adoption across a variety of domains reflects its remarkable potential and evolution. At the forefront of this cutting-edge research is Yongxin Chen, an associate professor in the Daniel Guggenheim School of Aerospace Engineering. He connects the power of machine learning, control, and robotics to develop more efficient and reliable AI systems.
When control and machine learning are applied to robotics, researchers and scientists can make more efficient robots that can have superior performance and outcomes. Through innovative studies, Chen’s work transforms the way humans interact with machines and advances the capabilities of autonomous systems.
(text and background only visible when logged in)
Algorithm Nation
Professor Chen conducts research in the Institute for Robotics and Intelligent Machines and the Machine Learning Center, both hubs for creating next generation robotics and AI.
“We’ve recently developed an algorithm used in diffusion models to generate AI samples faster,” he explained. Generative AI creates entirely new data that did not exist in the original training dataset. These data types can yield images, audio, text, and other forms of content. AI art is a common example, where a user puts in data that then generates a completely unique and new data sample in the form of a picture or image.
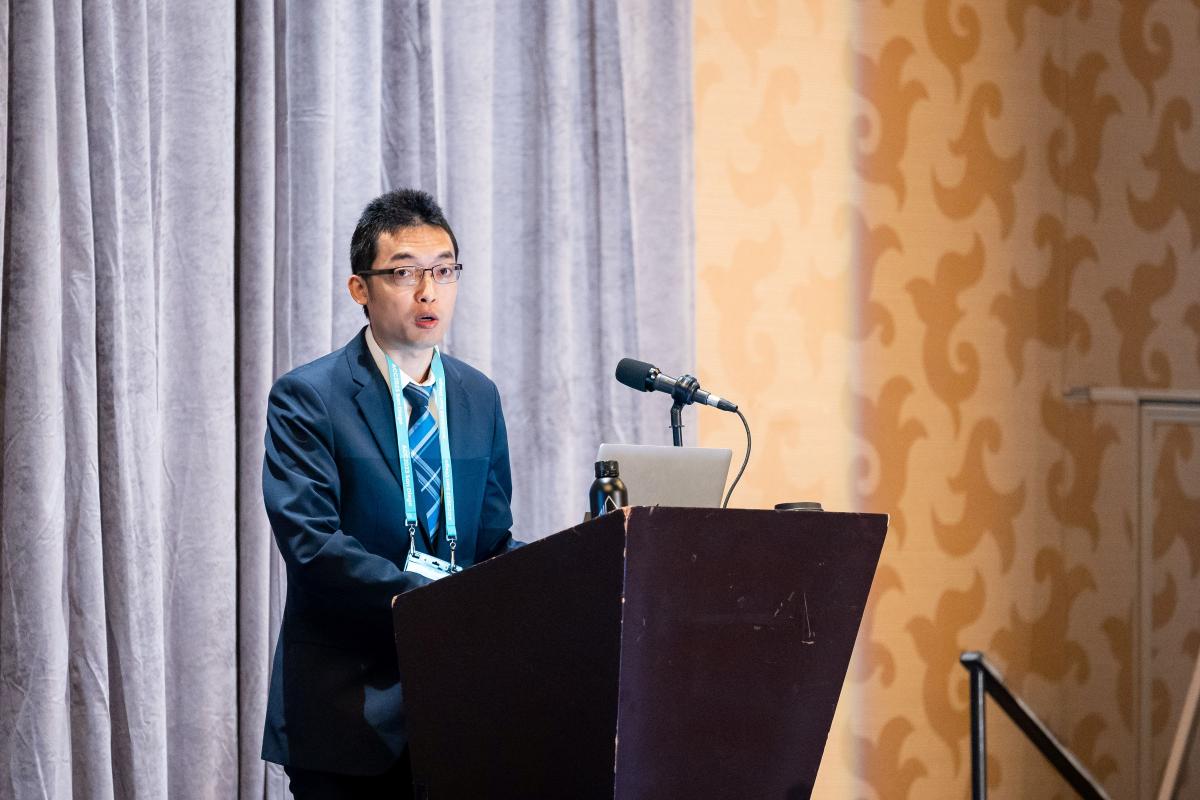
This type of data collection, needed for reliable research, can require supercomputing abilities due to the data size and file type. Acquiring and storing these datasets can be very expensive, inaccessible, and time consuming. Still, Chen’s lab has found some solutions.
“We recently developed a faster algorithm so that users can generate a picture faster. It improves the speed by a magnitude of 10 times faster than current capabilities. Thus, saving computational time and resources,” Chen explained.
“The data collecting in machine learning can be very expensive. This algorithm can rely on some available data and generate realistic data quickly and efficiently, without doing more experimenting, which can be very costly with autonomous vehicles, for example.”
Engineers can also use this type of technology to gather and augment data about Mars’ terrain, climate, and landscape to help vehicles land, travel, and takeoff safely on the red planet and ultimately learn more about a place that has yet to be explored by humans.
“This type of tool is critical because we cannot send human life to Mars right now, but we can send robots,” Chen explained.
Space exploration will be one step closer to that milestone with NASA’s Artemis II mission, planned for late-2024. Though the moon is about 238,855 miles from Earth and Mars is about 140 million miles from Earth, this mission will allow scientists to better understand long-term human life support systems, surface transportation systems, and space exploration.
Until then, AI will continue to be a tool to further test and run simulations using current data, something Chen thinks is an exciting aspect of his work.
(text and background only visible when logged in)
Robotics & Research
He found his affinity for robotics when he was a college student as a member of a robotics team where he and his teammates would design, build, and program robots to accomplish specific tasks and challenges. It was a rewarding experience for Chen which later led him to expand his engineering career to include research.
His research began in control theory, but expanded to include control, machine learning, and robotics. Control theory primarily deals with influencing different vehicles, or subjects, and uses systematic behavior to achieve a specific outcome.
For example, control theory enables drones to do three main things: maintain specific altitudes and positions by regulating the thrust of the motors; follow a specific path, following algorithms that will consider obstacles and deviations; and work harmoniously together in swarm operations while avoiding collusions. It plays a crucial role in achieving stability, precision, and efficiency in various engineering and real-world scenarios.
Machine learning is a natural fit for engineers because it allows robotics to understand data from cameras, lidar, radar, and other sensors to recognize patterns from the data collected, allowing robots to adapt to various obstacles and environments.
As a University of Minnesota (UMN) graduate student, he knew he wanted to pursue a career in engineering, but it hadn’t crossed his mind to become a professor. Having the passion and the natural ability to conduct research, he found his way to the classroom thanks to the direction of his Ph.D. advisor. Now, he is teaching Georgia Tech engineers while conducting meaningful research in a growing field.
I really enjoy interdisciplinary research. Conducting research at the intersection of control, machine learning, robotics, and other areas allows me to see important connections in these areas that are otherwise hidden. For instance, we’ve developed several control-inspired machine learning algorithms, which have state-of-the-art performance and designation.”
After receiving his doctoral degree in mechanical engineering, he spent one year as a postdoc at the Sloan Kettering Cancer Center in New York, where he performed medical imaging, which required analyzing and altering digital signals to make more accurate and reliable imaging. He later went on to Iowa State University, where he continued signal processing work while also working as an assistant professor, teaching electrical and computer engineering courses.
“This training and experiences are eye-opening for my career. They are important factors leading me to establish a research program at the intersection of control, machine learning, and robotics at Georgia Tech,” said Chen.
(text and background only visible when logged in)
(text and background only visible when logged in)
This training and experiences are eye-opening for my career. They are important factors leading me to establish a research program at the intersection of control, machine learning, and robotics at Georgia Tech.
Yongxin Chen
(text and background only visible when logged in)
Teaching the Next Generation
Today, he has three engineering courses in his teaching repertoire, one undergraduate-level, and two graduate-level courses. The graduate course - Optimal Transport Theory and Applications - he developed as an advanced-level course closely related to his research where students systematically go over optimal transport theory, while covering classical topics in optimal transport such as Kantorovich duality, Wasserstein distance, gradient flow, displacement interpolation.
He recently presented, as a plenary speaker, his research at the American Control Conference, one of the largest conferences in control, to discuss his journey through diffusions in control, inference, and learning.
“My long-term goal is to integrate model-based methods and data-driven methods for real physical world applications.”
(text and background only visible when logged in)
Related News
Yongxin Chen Receives the Donald P. Eckman Award
Yongxin Chen, assistant professor in the School of Aerospace Engineering, has been awarded the Donald P. Eckman Award from the American Automatic Control Council (AACC) for his work in stochastic control.
Professor Yongxin Chen and Team Win SIAM Best Paper
The Society for Industrial and Applied Mathematics (SIAM) has awarded the 2023 SIAM Activity Group on Control and Systems Theory Best SICON Paper Prize to Yongxin Chen and co-authors Isabel Haasler, Axel Ringh, and Johan Karlsson for their paper, ''Multimarginal Optimal Transport with a Tree-Structured Cost and the Schrödinger Bridge Problem.”